Use Cases mapped along the prostate cancer management continuum
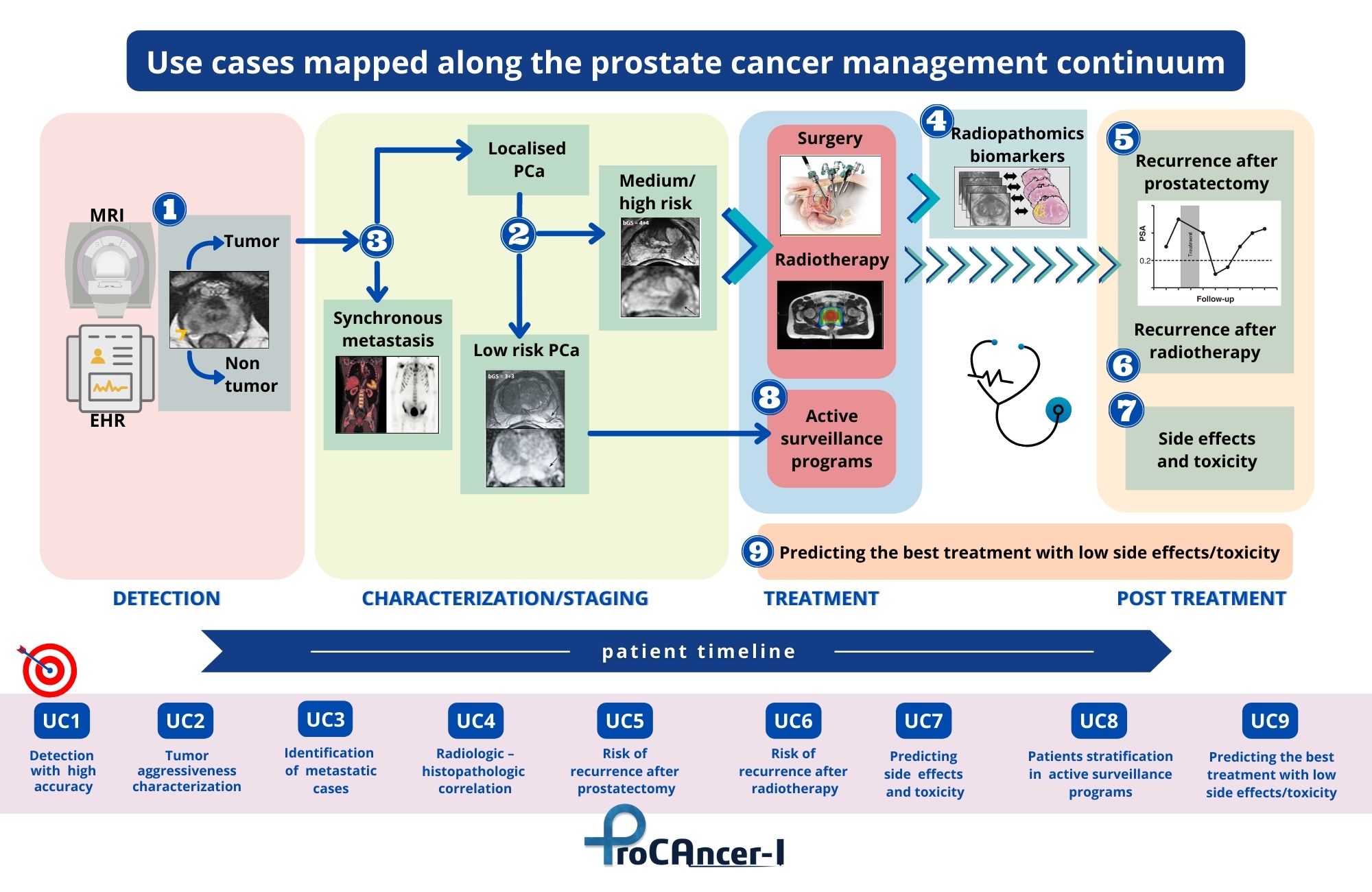
Nowadays, prostate cancer is highly curable if detected in its early stages. Indeed, when organ-confined at diagnosis, the five-year survival rate of prostate cancer is close to 100%. Unfortunately, current prostate cancer diagnostic pipeline has several limitations. Prostate specific antigen (PSA) has a low sensitivity and specificity. Approximately 15% of men with normal PSA values (below 4 ng/ml) will have prostate cancer, while in 75% of patients with PSA above 4 ng/ml the only findings will be prostate hypertrophy or inflammation. Moreover, when high values of PSA are detected in the blood of patients, biopsy is necessary to confirm diagnosis. Unfortunately, up to 30% of clinically significant tumours will be missed if a systematic approach is adopted for biopsy, i.e. sampling is performed randomly under ultrasound guidance.
When these tests fail to identify prostate cancer, patient’s long-term health and well-being might suffer an irreversible impact, because the later the diagnosis, the more likely that cancer will have grown and spread to remote parts of the body becoming a deadly disease. Hence, the main clinical challenges for the future is to identify prostate cancer with high accuracy, as early as possible, to stratify patients according to disease aggressiveness and to tailor therapy (or non-therapy) based on the risk of progression, comorbidities and life expectancy.
In this context, the Use Cases within the ProCAncer-I project represent the drivers to answer PCa relevant clinical questions, ranging from PCa diagnosis and characterization to prediction of treatment response and occurrence of side effects after treatment. The Use Cases were defined to create a unique dataset in terms of data quantity, quality and diversity, and to facilitate validation of the platform with its added value and usability for various users among the clinical and research community.
Use Cases mapped along the prostate cancer management continuum in the ProCAncer-I Project
In details, the 9 Use Cases identified are:
- Detection of prostate cancer with high accuracy both in peripheral and transitional zones to identify which men have cancer and those with no cancer. From a clinical point of view UC1 will help stratifying men with prostate hypertrophy or inflammation despite the high PSA values (>4 ng/ml) and those who should undergo additional diagnostic tests (e.g. biopsy) to identify if suspicious lesions identified on MRI are clinically significant or if there is an indolent disease with no harm for the patient;
- Characterization of cancer according to its biological aggressiveness into clinically significant and non-significant disease. UC2 aims to stratify men with suspicious findings on MRI into high-risk cases, which need radical treatments to ensure that cancer will not grow and spread to remote parts of the body becoming a deadly disease, from low-risk cases which could be safely followed-up with active surveillance, avoiding comorbidities of treatment and ensuring the highest possible quality of life for patients;
- Identification of patients with metastatic prostate cancer as early as possible among cases with high-risk PCa. Clinically, UC3 will help to adjust treatment strategies and mitigate metastatic spread that will finally kill the patient, as well as adjust follow up frequency to patients with high metastatic risk. AI models will provide early indications whether a patient belongs to the metastatic subtype that needs a different therapeutic approach, mining tumor characteristics that probably are related to its biological differences;
- Radiologic – Histopathologic correlation to provide biology-based validation of AI models to compare side by side pathologic data with AI results to improve understanding of the features that AI models are making use to reach specific decisions. Moreover, UC4 will help correlating imaging phenotype derived from MRI to microscopic findings from pathology and predicting cancer presence and/or its biology characteristics from radiologic imaging;
- Prediction of the risk of disease recurrence after radical prostatectomy, based on imaging data and AI techniques. In UC5 post-surgery findings, as positive surgical margins and extracapsular extension, will be considered in a nomogram comprising also radiomics and clinical variables to predict disease recurrence. UC5 will help clinicians to choose between different treatment techniques (conventional, nerve sparing, laparoscopic, robot-assisted radical prostatectomy), tailoring treatment to the predicted risk of disease recurrence;
- Prediction of treatment response in case of radiation therapy, assessing the risk of disease recurrence to promptly adjust therapeutic strategy at an early stage and avoid patient discomfort and non-optimal distribution of medical resources. UC6 is similar to UC5, but refers to radiotherapy recurrence and it will help radiation oncologist to tailor treatments;
- Prediction of post radical prostatectomy and/or radiation-induced urinary toxicity, in order to consider additional or alternative measures to alleviate therapy-induced undesired effects. Using UC7 results, patients with high risk for toxicity can be thoroughly informed on the side effects and alternative possibilities for therapy. This could help balancing the risk-benefit ratio related to whole gland treatments, in particular in patients with no life-threatening PCa. UC7 will take into consideration urinary incontinence, irritative/obstructive bowel, sexual/erectile dysfunction, and hormonal domains;
- AI-powered patient stratification for enrolment in Active Surveillance programs, to develop a more efficient patient stratification program based on AI decision-making from MRI lesion phenotype. The risk of disease progression in patients who are undergoing active surveillance will be assessed with longitudinal MRI data (combined with biopsy) to reach specific clinical indications (either repeat PSA test, MRI, biopsy or a combination of them). UC8 aims also to predict the time‑to‑progress to provide a follow-up strategy and stratify patients in those who could safely remain in the active surveillance group and those who will ultimately need treatment;
- Prediction of the best option for patients needing treatment ensuring the lowest possible side effects/toxicity. Results from all previous Use Cases are expected to merge into a holistic model suggesting presence/non-presence of PCa, stratification into clinically significant/insignificant cases and a decision support system suggesting the best treatment option (radical prostatectomy, radiation therapy, active surveillance), considering also the lowest toxicity/side effects to ensure the best possible quality of life.
Summary of the estimated number of subjects included in each single use case. UC = use case; RP = radical prostatectomy; RT = radiation therapy; AS = active surveillance; MTS = metastatic disease